数值分析-函数逼近&&插值、函数逼近&曲线拟合的区别
一、注意插值、函数逼近、曲线拟合的区别:
插值:没有原函数概念,是给定一些点,要求出一个简单函数,使得该简单函数通过所有点,或者更进一步,要求简单函数的一阶、二阶导数等于某些值或者分段插值时在分段点的左右一阶、二阶导数相等,或者还有其他条件等等。
函数逼近:已知原函数的情况下,找一个简单函数逼近于原函数,使得在定义域上的某个点的误差达到最小(这个点是误差达到最大值的点)。
曲线拟合:原函数未知,给定原函数的若干点,在某个简单函数空间找出使得总误差(根据不同定义有不同的误差距离的衡量,一般是均方误差)最小的那个简单函数。而且这个函数要根据特定的问题设计特定的形式。
二、函数逼近
这里主要讲多项式的逼近方法。
1、正交多项式
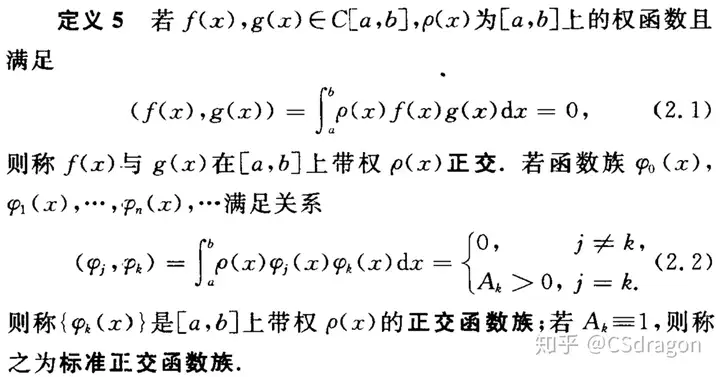
其中权函数定义:
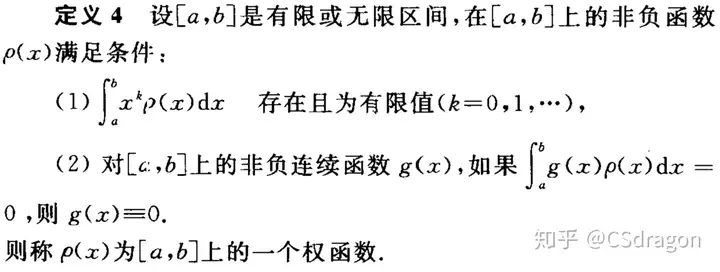
正交多项式的定义:
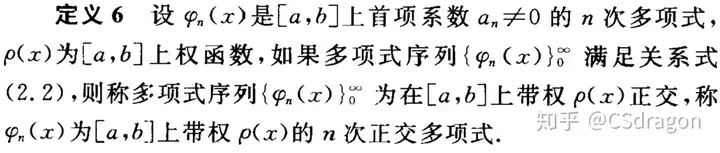
其中 {φ(xn)}0∞\left\{ \varphi(x_{n}) \right\}_{0}^{\infty}表示所有线性无关的多项式生成的无限维线性空间。
正交多项式的性质:
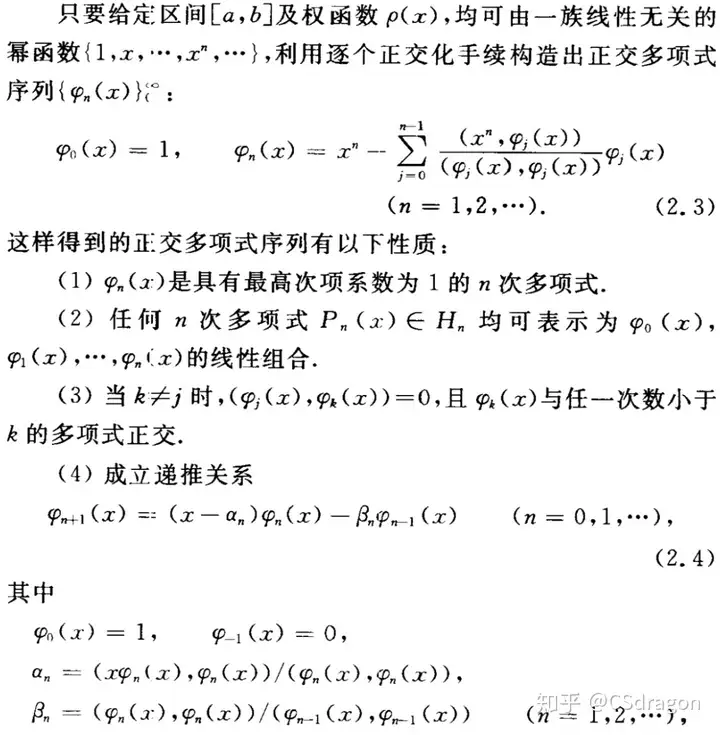

2、勒让德正交多项式
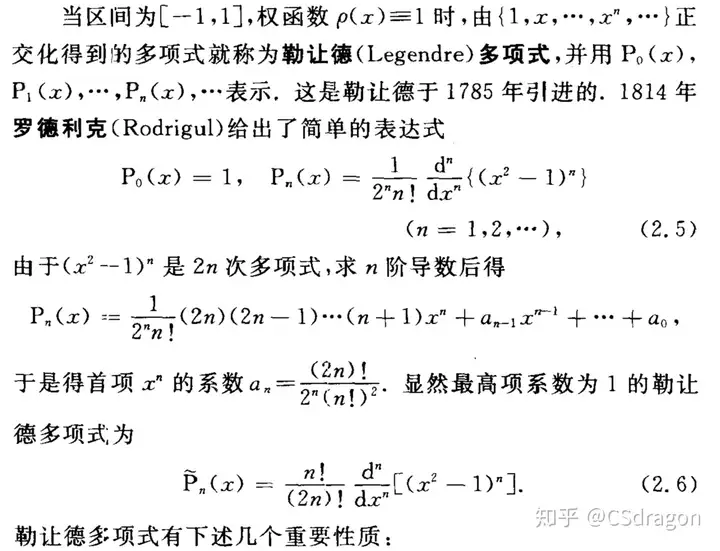
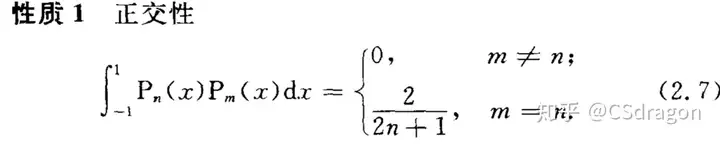
性质2 :奇偶性

性质3:递推关系


2、切比雪夫正交多项式
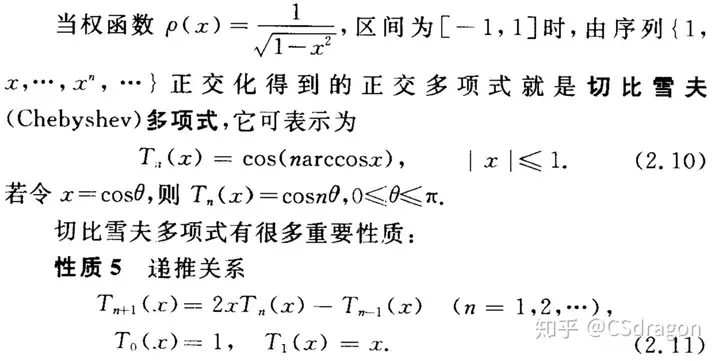
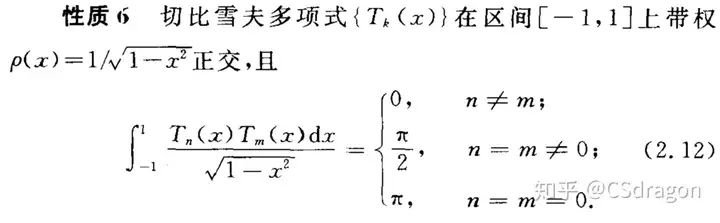
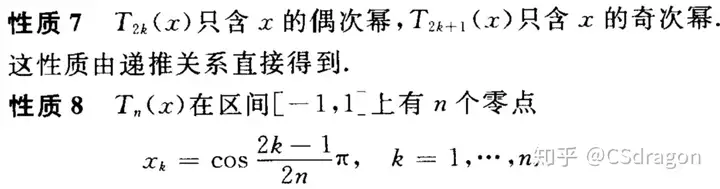
根据选取的权函数不同,由 {x,x2,...,xn,...}\left\{ x, x_{2}, ..., x_{n}, ... \right\} 正交化得到不同的正交化多项式。
3、最佳一致逼近多项式
误差定义:

最佳一致逼近多项式定义:可以证明最佳一致逼近多项式是一定存在的。在最佳一致逼近多项式中误差达到最大的点叫做偏差点。
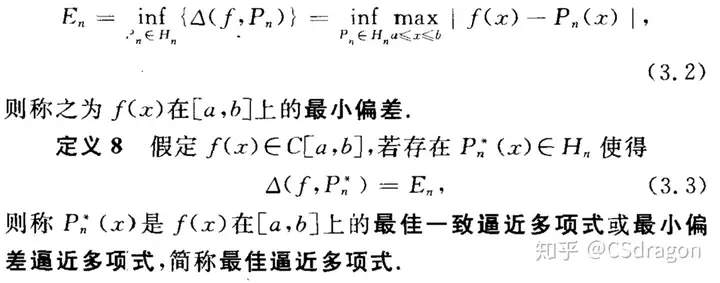
最佳一致逼近多项式的充要条件:



一般情况下用定理五计算P(x)比较难,应用定理六解决一些简单问题:
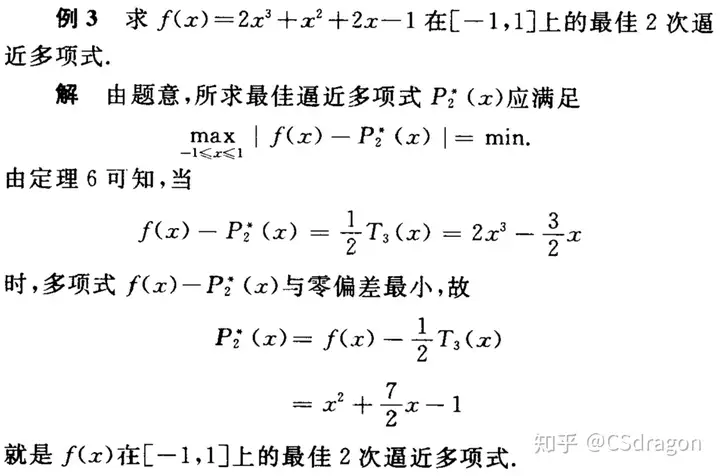
4、最佳平方逼近
定义:
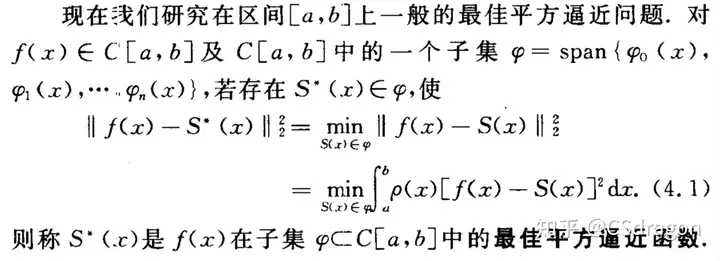
推导过程:(4.1)问题等价于求 I(a0,a1,...,an)I\left( a_{0}, a_{1}, ..., a_{n} \right) 的最小值。
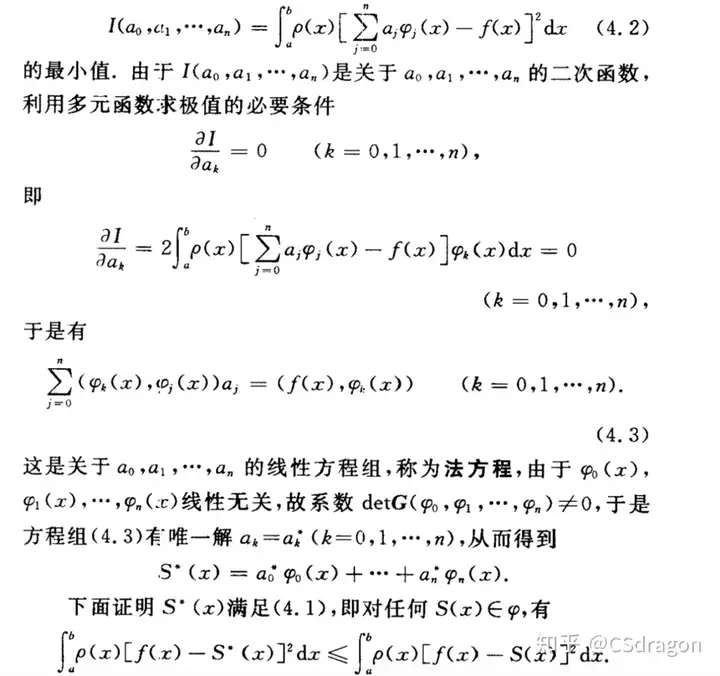
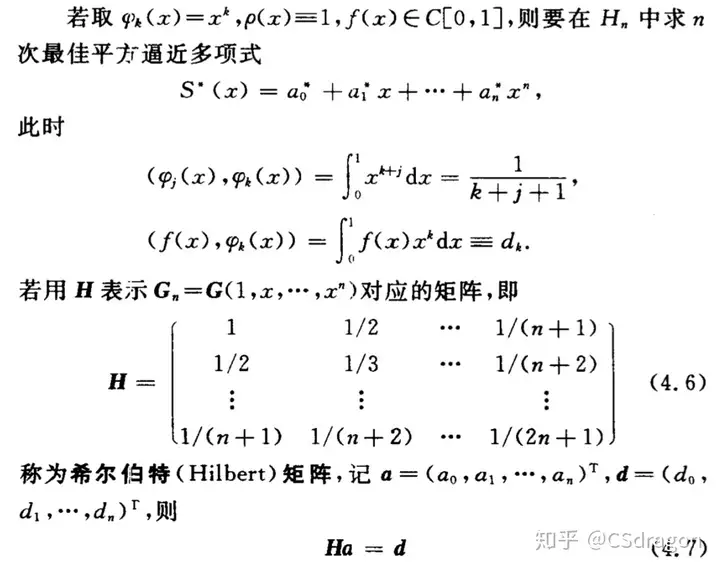
举个例子:
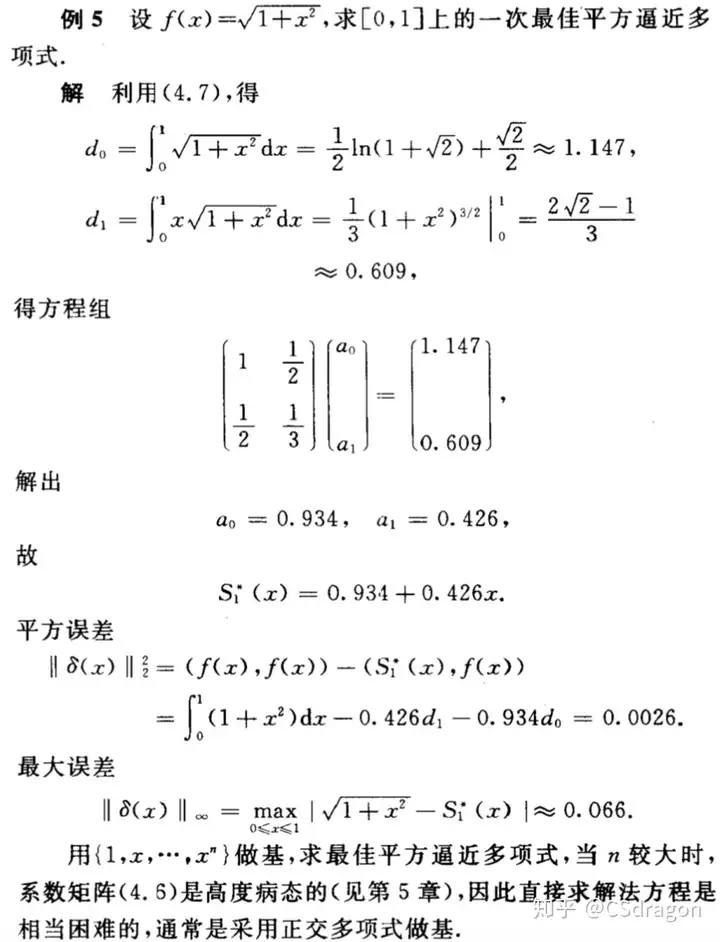
本站所有文章、数据、图片均来自互联网,一切版权均归源网站或源作者所有。
如果侵犯了你的权益请来信告知我们删除。邮箱:dacesmiling@qq.com